
Keras Cheat Sheet: Neural Networks in Python Make your own neural networks with this Keras cheat sheet to deep learning in Python for beginners, with code samples. Keras is an easy-to-use and powerful library for Theano and TensorFlow that provides a high-level neural networks API to develop and evaluate deep learning models. Keras Cheat Sheet Numpy. NumPy targets the CPython reference implementation of Python, which is a non-optimizing bytecode interpreter. Mathematical algorithms written. This is a huge Data Science cheat sheet. Thanks for taking the time to help us. I consider this post one of the best for learning and have near!!👍. Keras Cheat Sheet: Deep Learning in Python - Sep 27, 2017. Keras is a Python deep learning library for Theano and TensorFlow. The package is easy to use and powerful, as it provides users with a high-level neural networks API to develop and evaluate deep learning models.
By Afshine Amidi and Shervine Amidi
Overview
Architecture of a traditional CNN Convolutional neural networks, also known as CNNs, are a specific type of neural networks that are generally composed of the following layers:
The convolution layer and the pooling layer can be fine-tuned with respect to hyperparameters that are described in the next sections.
Types of layer
Convolution layer (CONV) The convolution layer (CONV) uses filters that perform convolution operations as it is scanning the input $I$ with respect to its dimensions. Its hyperparameters include the filter size $F$ and stride $S$. The resulting output $O$ is called feature map or activation map.

Tensorflow Cheat Sheet Pdf
Remark: the convolution step can be generalized to the 1D and 3D cases as well.
Pooling (POOL) The pooling layer (POOL) is a downsampling operation, typically applied after a convolution layer, which does some spatial invariance. In particular, max and average pooling are special kinds of pooling where the maximum and average value is taken, respectively.
Type | Max pooling | Average pooling |
Purpose | Each pooling operation selects the maximum value of the current view | Each pooling operation averages the values of the current view |
Illustration | ||
Comments | • Preserves detected features • Most commonly used | • Downsamples feature map • Used in LeNet |
Fully Connected (FC) The fully connected layer (FC) operates on a flattened input where each input is connected to all neurons. If present, FC layers are usually found towards the end of CNN architectures and can be used to optimize objectives such as class scores.
Filter hyperparameters
The convolution layer contains filters for which it is important to know the meaning behind its hyperparameters.
Dimensions of a filter A filter of size $Ftimes F$ applied to an input containing $C$ channels is a $F times F times C$ volume that performs convolutions on an input of size $I times I times C$ and produces an output feature map (also called activation map) of size $O times O times 1$.
Remark: the application of $K$ filters of size $Ftimes F$ results in an output feature map of size $O times O times K$.
Stride For a convolutional or a pooling operation, the stride $S$ denotes the number of pixels by which the window moves after each operation.
Zero-padding Zero-padding denotes the process of adding $P$ zeroes to each side of the boundaries of the input. This value can either be manually specified or automatically set through one of the three modes detailed below:
Mode | Valid | Same | Full |
Value | $P = 0$ | $P_text{start} = Bigllfloorfrac{S lceilfrac{I}{S}rceil - I + F - S}{2}Bigrrfloor$ $P_text{end} = Bigllceilfrac{S lceilfrac{I}{S}rceil - I + F - S}{2}Bigrrceil$ | $P_text{start}in[![0,F-1]!]$ $P_text{end} = F-1$ |
Illustration | |||
Purpose | • No padding • Drops last convolution if dimensions do not match | • Padding such that feature map size has size $Bigllceilfrac{I}{S}Bigrrceil$ • Output size is mathematically convenient • Also called 'half' padding | • Maximum padding such that end convolutions are applied on the limits of the input • Filter 'sees' the input end-to-end |
Tuning hyperparameters
Parameter compatibility in convolution layer By noting $I$ the length of the input volume size, $F$ the length of the filter, $P$ the amount of zero padding, $S$ the stride, then the output size $O$ of the feature map along that dimension is given by:
Remark: often times, $P_text{start} = P_text{end} triangleq P$, in which case we can replace $P_text{start} + P_text{end}$ by $2P$ in the formula above.
Understanding the complexity of the model In order to assess the complexity of a model, it is often useful to determine the number of parameters that its architecture will have. In a given layer of a convolutional neural network, it is done as follows:
CONV | POOL | FC | |
Illustration | |||
Input size | $I times I times C$ | $I times I times C$ | $N_{text{in}}$ |
Output size | $O times O times K$ | $O times O times C$ | $N_{text{out}}$ |
Number of parameters | $(F times F times C + 1) cdot K$ | $0$ | $(N_{text{in}} + 1 ) times N_{text{out}}$ |
Remarks | • One bias parameter per filter • In most cases, $S < F$ • A common choice for $K$ is $2C$ | • Pooling operation done channel-wise • In most cases, $S = F$ | • Input is flattened • One bias parameter per neuron • The number of FC neurons is free of structural constraints |
Receptive field The receptive field at layer $k$ is the area denoted $R_k times R_k$ of the input that each pixel of the $k$-th activation map can 'see'.By calling $F_j$ the filter size of layer $j$ and $S_i$ the stride value of layer $i$ and with the convention $S_0 = 1$, the receptive field at layer $k$ can be computed with the formula:
In the example below, we have $F_1 = F_2 = 3$ and $S_1 = S_2 = 1$, which gives $R_2 = 1 + 2cdot 1 + 2cdot 1 = 5$.
Commonly used activation functions
Rectified Linear Unit The rectified linear unit layer (ReLU) is an activation function $g$ that is used on all elements of the volume. It aims at introducing non-linearities to the network. Its variants are summarized in the table below:
ReLU | Leaky ReLU | ELU |
$g(z)=max(0,z)$ | $g(z)=max(epsilon z,z)$ with $epsilonll1$ | $g(z)=max(alpha(e^z-1),z)$ with $alphall1$ |
• Non-linearity complexities biologically interpretable | • Addresses dying ReLU issue for negative values | • Differentiable everywhere |
Softmax The softmax step can be seen as a generalized logistic function that takes as input a vector of scores $xinmathbb{R}^n$ and outputs a vector of output probability $pinmathbb{R}^n$ through a softmax function at the end of the architecture. It is defined as follows:
Object detection
Types of models There are 3 main types of object recognition algorithms, for which the nature of what is predicted is different. They are described in the table below:
Image classification | Classification w. localization | Detection |
• Classifies a picture • Predicts probability of object | • Detects an object in a picture • Predicts probability of object and where it is located | • Detects up to several objects in a picture • Predicts probabilities of objects and where they are located |
Traditional CNN | Simplified YOLO, R-CNN | YOLO, R-CNN |
Detection In the context of object detection, different methods are used depending on whether we just want to locate the object or detect a more complex shape in the image. The two main ones are summed up in the table below:
Bounding box detection | Landmark detection |
• Detects the part of the image where the object is located | • Detects a shape or characteristics of an object (e.g. eyes) • More granular |
Box of center $(b_x,b_y)$, height $b_h$ and width $b_w$ | Reference points $(l_{1x},l_{1y}),$ $...,$ $(l_{nx},l_{ny})$ |
Machine Learning Cheat Sheet
Intersection over Union Intersection over Union, also known as $textrm{IoU}$, is a function that quantifies how correctly positioned a predicted bounding box $B_p$ is over the actual bounding box $B_a$. It is defined as:
Remark: we always have $textrm{IoU}in[0,1]$. By convention, a predicted bounding box $B_p$ is considered as being reasonably good if $textrm{IoU}(B_p,B_a)geqslant0.5$.
Anchor boxes Anchor boxing is a technique used to predict overlapping bounding boxes. In practice, the network is allowed to predict more than one box simultaneously, where each box prediction is constrained to have a given set of geometrical properties. For instance, the first prediction can potentially be a rectangular box of a given form, while the second will be another rectangular box of a different geometrical form.
Non-max suppression The non-max suppression technique aims at removing duplicate overlapping bounding boxes of a same object by selecting the most representative ones. After having removed all boxes having a probability prediction lower than 0.6, the following steps are repeated while there are boxes remaining:
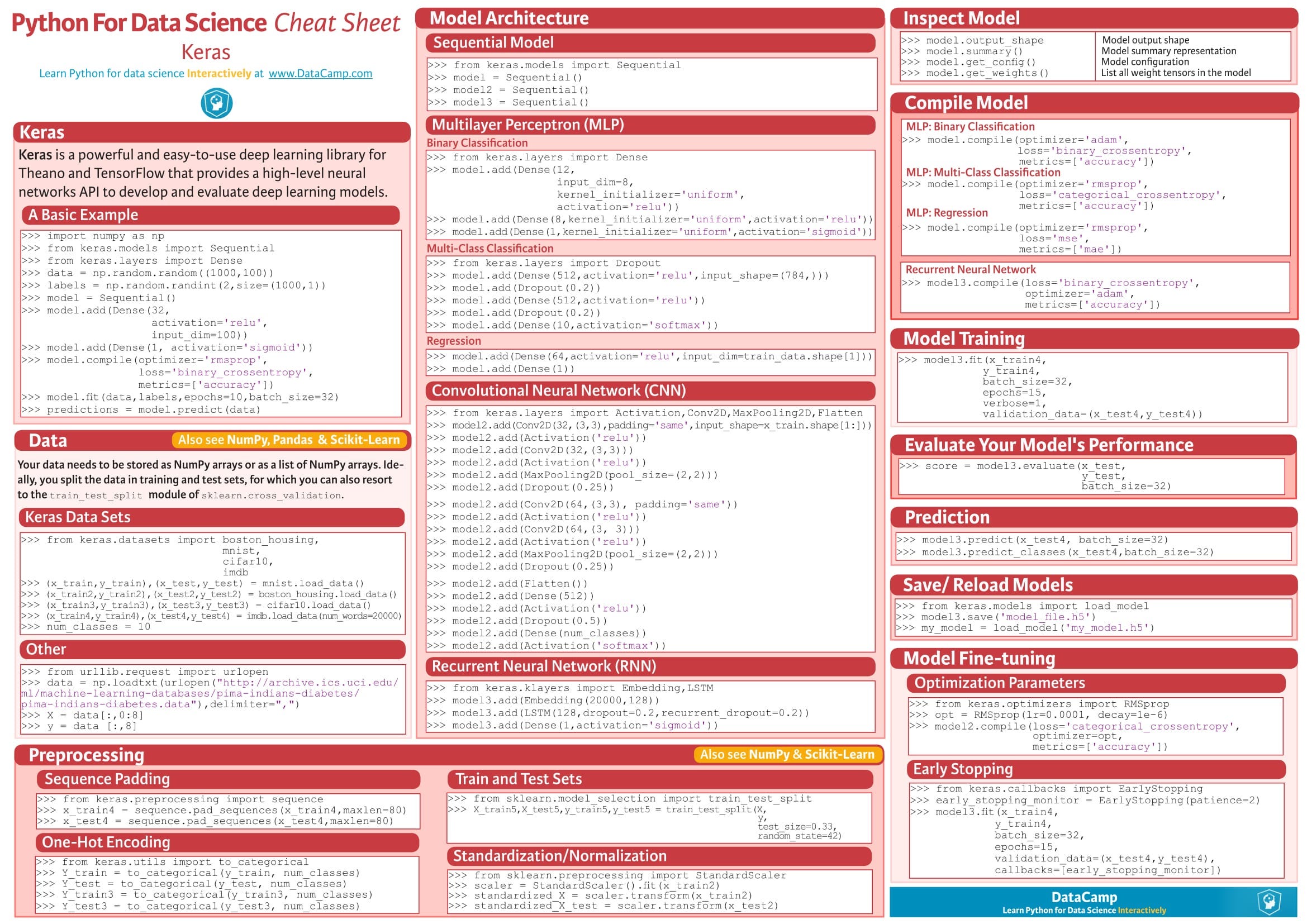
For a given class,
• Step 1: Pick the box with the largest prediction probability.
• Step 2: Discard any box having an $textrm{IoU}geqslant0.5$ with the previous box.
YOLO You Only Look Once (YOLO) is an object detection algorithm that performs the following steps:
• Step 1: Divide the input image into a $Gtimes G$ grid.
• Step 2: For each grid cell, run a CNN that predicts $y$ of the following form:
• Step 3: Run the non-max suppression algorithm to remove any potential duplicate overlapping bounding boxes.
Remark: when $p_c=0$, then the network does not detect any object. In that case, the corresponding predictions $b_x, ..., c_p$ have to be ignored.
R-CNN Region with Convolutional Neural Networks (R-CNN) is an object detection algorithm that first segments the image to find potential relevant bounding boxes and then run the detection algorithm to find most probable objects in those bounding boxes.
Remark: although the original algorithm is computationally expensive and slow, newer architectures enabled the algorithm to run faster, such as Fast R-CNN and Faster R-CNN.
Face verification and recognition
Types of models Two main types of model are summed up in table below:
Face verification | Face recognition |
• Is this the correct person? • One-to-one lookup | • Is this one of the $K$ persons in the database? • One-to-many lookup |
One Shot Learning One Shot Learning is a face verification algorithm that uses a limited training set to learn a similarity function that quantifies how different two given images are. The similarity function applied to two images is often noted $d(textrm{image 1}, textrm{image 2}).$
Siamese Network Siamese Networks aim at learning how to encode images to then quantify how different two images are. For a given input image $x^{(i)}$, the encoded output is often noted as $f(x^{(i)})$.
Triplet loss The triplet loss $ell$ is a loss function computed on the embedding representation of a triplet of images $A$ (anchor), $P$ (positive) and $N$ (negative). The anchor and the positive example belong to a same class, while the negative example to another one. By calling $alphainmathbb{R}^+$ the margin parameter, this loss is defined as follows:
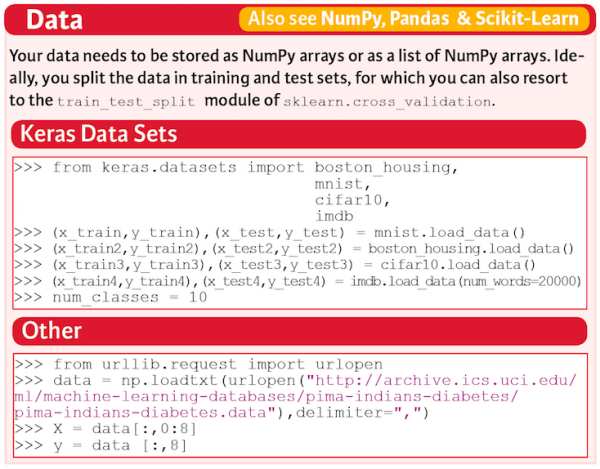
Neural style transfer
Motivation The goal of neural style transfer is to generate an image $G$ based on a given content $C$ and a given style $S$.
Activation In a given layer $l$, the activation is noted $a^{[l]}$ and is of dimensions $n_Htimes n_wtimes n_c$
Content cost function The content cost function $J_{textrm{content}}(C,G)$ is used to determine how the generated image $G$ differs from the original content image $C$. It is defined as follows:
Style matrix The style matrix $G^{[l]}$ of a given layer $l$ is a Gram matrix where each of its elements $G_{kk'}^{[l]}$ quantifies how correlated the channels $k$ and $k'$ are. It is defined with respect to activations $a^{[l]}$ as follows:
Remark: the style matrix for the style image and the generated image are noted $G^{[l](S)}$ and $G^{[l](G)}$ respectively.
Style cost function The style cost function $J_{textrm{style}}(S,G)$ is used to determine how the generated image $G$ differs from the style $S$. It is defined as follows:
Overall cost function The overall cost function is defined as being a combination of the content and style cost functions, weighted by parameters $alpha,beta$, as follows:
Remark: a higher value of $alpha$ will make the model care more about the content while a higher value of $beta$ will make it care more about the style.
Architectures using computational tricks
Generative Adversarial Network Generative adversarial networks, also known as GANs, are composed of a generative and a discriminative model, where the generative model aims at generating the most truthful output that will be fed into the discriminative which aims at differentiating the generated and true image.
Remark: use cases using variants of GANs include text to image, music generation and synthesis.
ResNet The Residual Network architecture (also called ResNet) uses residual blocks with a high number of layers meant to decrease the training error. The residual block has the following characterizing equation:
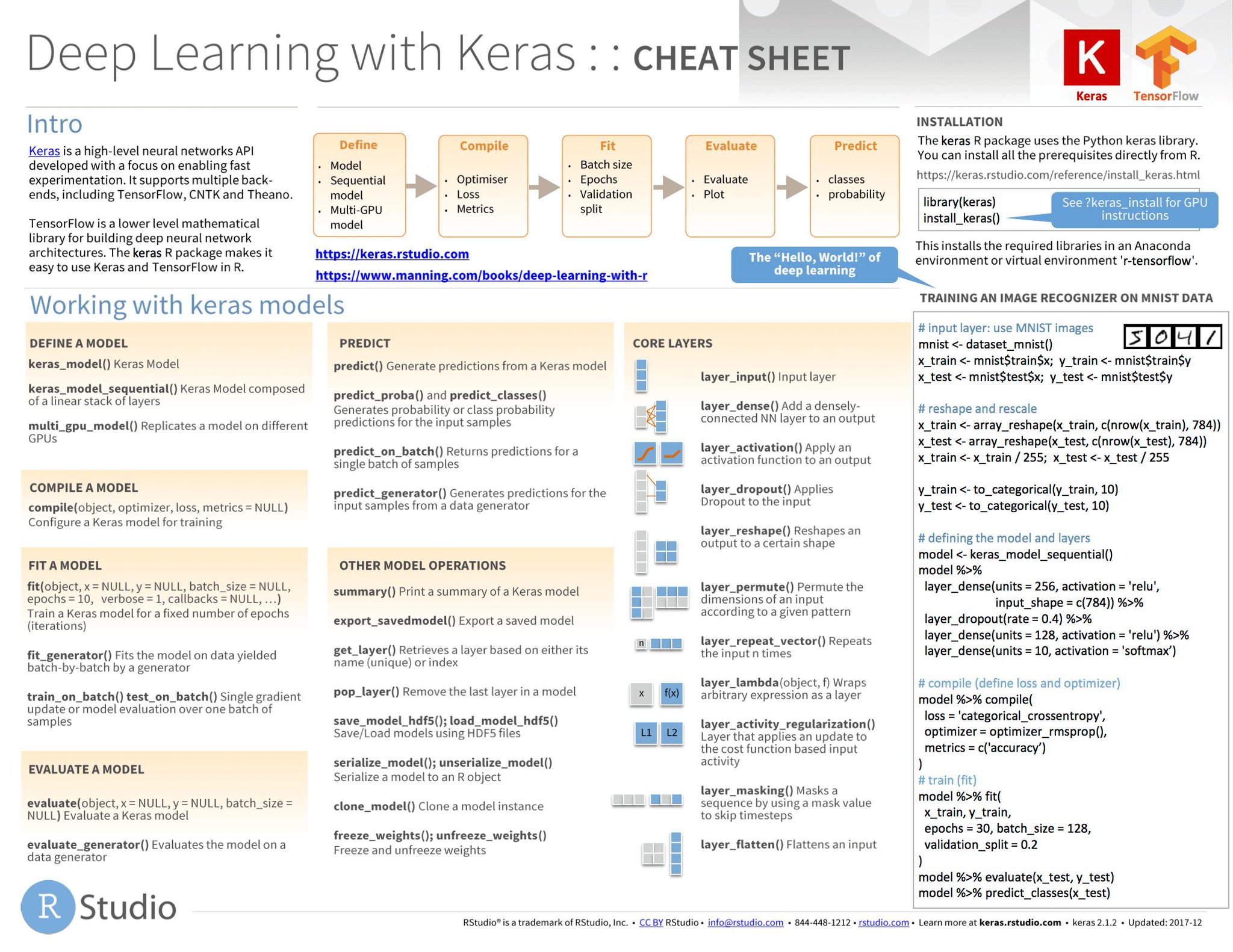
Keras Cheat Sheet: Neural Networks in Python Make your own neural networks with this Keras cheat sheet to deep learning in Python for beginners, with code samples. Keras is an easy-to-use and powerful library for Theano and TensorFlow that provides a high-level neural networks API to develop and evaluate deep learning models. Keras Cheat Sheet Numpy. NumPy targets the CPython reference implementation of Python, which is a non-optimizing bytecode interpreter. Mathematical algorithms written. This is a huge Data Science cheat sheet. Thanks for taking the time to help us. I consider this post one of the best for learning and have near!!👍. Keras Cheat Sheet: Deep Learning in Python - Sep 27, 2017. Keras is a Python deep learning library for Theano and TensorFlow. The package is easy to use and powerful, as it provides users with a high-level neural networks API to develop and evaluate deep learning models.
By Afshine Amidi and Shervine Amidi
Overview
Architecture of a traditional CNN Convolutional neural networks, also known as CNNs, are a specific type of neural networks that are generally composed of the following layers:
The convolution layer and the pooling layer can be fine-tuned with respect to hyperparameters that are described in the next sections.
Types of layer
Convolution layer (CONV) The convolution layer (CONV) uses filters that perform convolution operations as it is scanning the input $I$ with respect to its dimensions. Its hyperparameters include the filter size $F$ and stride $S$. The resulting output $O$ is called feature map or activation map.
Tensorflow Cheat Sheet Pdf
Remark: the convolution step can be generalized to the 1D and 3D cases as well.
Pooling (POOL) The pooling layer (POOL) is a downsampling operation, typically applied after a convolution layer, which does some spatial invariance. In particular, max and average pooling are special kinds of pooling where the maximum and average value is taken, respectively.
Type | Max pooling | Average pooling |
Purpose | Each pooling operation selects the maximum value of the current view | Each pooling operation averages the values of the current view |
Illustration | ||
Comments | • Preserves detected features • Most commonly used | • Downsamples feature map • Used in LeNet |
Fully Connected (FC) The fully connected layer (FC) operates on a flattened input where each input is connected to all neurons. If present, FC layers are usually found towards the end of CNN architectures and can be used to optimize objectives such as class scores.
Filter hyperparameters
The convolution layer contains filters for which it is important to know the meaning behind its hyperparameters.
Dimensions of a filter A filter of size $Ftimes F$ applied to an input containing $C$ channels is a $F times F times C$ volume that performs convolutions on an input of size $I times I times C$ and produces an output feature map (also called activation map) of size $O times O times 1$.
Remark: the application of $K$ filters of size $Ftimes F$ results in an output feature map of size $O times O times K$.
Stride For a convolutional or a pooling operation, the stride $S$ denotes the number of pixels by which the window moves after each operation.
Zero-padding Zero-padding denotes the process of adding $P$ zeroes to each side of the boundaries of the input. This value can either be manually specified or automatically set through one of the three modes detailed below:
Mode | Valid | Same | Full |
Value | $P = 0$ | $P_text{start} = Bigllfloorfrac{S lceilfrac{I}{S}rceil - I + F - S}{2}Bigrrfloor$ $P_text{end} = Bigllceilfrac{S lceilfrac{I}{S}rceil - I + F - S}{2}Bigrrceil$ | $P_text{start}in[![0,F-1]!]$ $P_text{end} = F-1$ |
Illustration | |||
Purpose | • No padding • Drops last convolution if dimensions do not match | • Padding such that feature map size has size $Bigllceilfrac{I}{S}Bigrrceil$ • Output size is mathematically convenient • Also called 'half' padding | • Maximum padding such that end convolutions are applied on the limits of the input • Filter 'sees' the input end-to-end |
Tuning hyperparameters
Parameter compatibility in convolution layer By noting $I$ the length of the input volume size, $F$ the length of the filter, $P$ the amount of zero padding, $S$ the stride, then the output size $O$ of the feature map along that dimension is given by:
Remark: often times, $P_text{start} = P_text{end} triangleq P$, in which case we can replace $P_text{start} + P_text{end}$ by $2P$ in the formula above.
Understanding the complexity of the model In order to assess the complexity of a model, it is often useful to determine the number of parameters that its architecture will have. In a given layer of a convolutional neural network, it is done as follows:
CONV | POOL | FC | |
Illustration | |||
Input size | $I times I times C$ | $I times I times C$ | $N_{text{in}}$ |
Output size | $O times O times K$ | $O times O times C$ | $N_{text{out}}$ |
Number of parameters | $(F times F times C + 1) cdot K$ | $0$ | $(N_{text{in}} + 1 ) times N_{text{out}}$ |
Remarks | • One bias parameter per filter • In most cases, $S < F$ • A common choice for $K$ is $2C$ | • Pooling operation done channel-wise • In most cases, $S = F$ | • Input is flattened • One bias parameter per neuron • The number of FC neurons is free of structural constraints |
Receptive field The receptive field at layer $k$ is the area denoted $R_k times R_k$ of the input that each pixel of the $k$-th activation map can 'see'.By calling $F_j$ the filter size of layer $j$ and $S_i$ the stride value of layer $i$ and with the convention $S_0 = 1$, the receptive field at layer $k$ can be computed with the formula:
In the example below, we have $F_1 = F_2 = 3$ and $S_1 = S_2 = 1$, which gives $R_2 = 1 + 2cdot 1 + 2cdot 1 = 5$.
Commonly used activation functions
Rectified Linear Unit The rectified linear unit layer (ReLU) is an activation function $g$ that is used on all elements of the volume. It aims at introducing non-linearities to the network. Its variants are summarized in the table below:
ReLU | Leaky ReLU | ELU |
$g(z)=max(0,z)$ | $g(z)=max(epsilon z,z)$ with $epsilonll1$ | $g(z)=max(alpha(e^z-1),z)$ with $alphall1$ |
• Non-linearity complexities biologically interpretable | • Addresses dying ReLU issue for negative values | • Differentiable everywhere |
Softmax The softmax step can be seen as a generalized logistic function that takes as input a vector of scores $xinmathbb{R}^n$ and outputs a vector of output probability $pinmathbb{R}^n$ through a softmax function at the end of the architecture. It is defined as follows:
Object detection
Types of models There are 3 main types of object recognition algorithms, for which the nature of what is predicted is different. They are described in the table below:
Image classification | Classification w. localization | Detection |
• Classifies a picture • Predicts probability of object | • Detects an object in a picture • Predicts probability of object and where it is located | • Detects up to several objects in a picture • Predicts probabilities of objects and where they are located |
Traditional CNN | Simplified YOLO, R-CNN | YOLO, R-CNN |
Detection In the context of object detection, different methods are used depending on whether we just want to locate the object or detect a more complex shape in the image. The two main ones are summed up in the table below:
Bounding box detection | Landmark detection |
• Detects the part of the image where the object is located | • Detects a shape or characteristics of an object (e.g. eyes) • More granular |
Box of center $(b_x,b_y)$, height $b_h$ and width $b_w$ | Reference points $(l_{1x},l_{1y}),$ $...,$ $(l_{nx},l_{ny})$ |
Machine Learning Cheat Sheet
Intersection over Union Intersection over Union, also known as $textrm{IoU}$, is a function that quantifies how correctly positioned a predicted bounding box $B_p$ is over the actual bounding box $B_a$. It is defined as:
Remark: we always have $textrm{IoU}in[0,1]$. By convention, a predicted bounding box $B_p$ is considered as being reasonably good if $textrm{IoU}(B_p,B_a)geqslant0.5$.
Anchor boxes Anchor boxing is a technique used to predict overlapping bounding boxes. In practice, the network is allowed to predict more than one box simultaneously, where each box prediction is constrained to have a given set of geometrical properties. For instance, the first prediction can potentially be a rectangular box of a given form, while the second will be another rectangular box of a different geometrical form.
Non-max suppression The non-max suppression technique aims at removing duplicate overlapping bounding boxes of a same object by selecting the most representative ones. After having removed all boxes having a probability prediction lower than 0.6, the following steps are repeated while there are boxes remaining:
For a given class,
• Step 1: Pick the box with the largest prediction probability.
• Step 2: Discard any box having an $textrm{IoU}geqslant0.5$ with the previous box.
YOLO You Only Look Once (YOLO) is an object detection algorithm that performs the following steps:
• Step 1: Divide the input image into a $Gtimes G$ grid.
• Step 2: For each grid cell, run a CNN that predicts $y$ of the following form:
• Step 3: Run the non-max suppression algorithm to remove any potential duplicate overlapping bounding boxes.
Remark: when $p_c=0$, then the network does not detect any object. In that case, the corresponding predictions $b_x, ..., c_p$ have to be ignored.
R-CNN Region with Convolutional Neural Networks (R-CNN) is an object detection algorithm that first segments the image to find potential relevant bounding boxes and then run the detection algorithm to find most probable objects in those bounding boxes.
Remark: although the original algorithm is computationally expensive and slow, newer architectures enabled the algorithm to run faster, such as Fast R-CNN and Faster R-CNN.
Face verification and recognition
Types of models Two main types of model are summed up in table below:
Face verification | Face recognition |
• Is this the correct person? • One-to-one lookup | • Is this one of the $K$ persons in the database? • One-to-many lookup |
One Shot Learning One Shot Learning is a face verification algorithm that uses a limited training set to learn a similarity function that quantifies how different two given images are. The similarity function applied to two images is often noted $d(textrm{image 1}, textrm{image 2}).$
Siamese Network Siamese Networks aim at learning how to encode images to then quantify how different two images are. For a given input image $x^{(i)}$, the encoded output is often noted as $f(x^{(i)})$.
Triplet loss The triplet loss $ell$ is a loss function computed on the embedding representation of a triplet of images $A$ (anchor), $P$ (positive) and $N$ (negative). The anchor and the positive example belong to a same class, while the negative example to another one. By calling $alphainmathbb{R}^+$ the margin parameter, this loss is defined as follows:
Neural style transfer
Motivation The goal of neural style transfer is to generate an image $G$ based on a given content $C$ and a given style $S$.
Activation In a given layer $l$, the activation is noted $a^{[l]}$ and is of dimensions $n_Htimes n_wtimes n_c$
Content cost function The content cost function $J_{textrm{content}}(C,G)$ is used to determine how the generated image $G$ differs from the original content image $C$. It is defined as follows:
Style matrix The style matrix $G^{[l]}$ of a given layer $l$ is a Gram matrix where each of its elements $G_{kk'}^{[l]}$ quantifies how correlated the channels $k$ and $k'$ are. It is defined with respect to activations $a^{[l]}$ as follows:
Remark: the style matrix for the style image and the generated image are noted $G^{[l](S)}$ and $G^{[l](G)}$ respectively.
Style cost function The style cost function $J_{textrm{style}}(S,G)$ is used to determine how the generated image $G$ differs from the style $S$. It is defined as follows:
Overall cost function The overall cost function is defined as being a combination of the content and style cost functions, weighted by parameters $alpha,beta$, as follows:
Remark: a higher value of $alpha$ will make the model care more about the content while a higher value of $beta$ will make it care more about the style.
Architectures using computational tricks
Generative Adversarial Network Generative adversarial networks, also known as GANs, are composed of a generative and a discriminative model, where the generative model aims at generating the most truthful output that will be fed into the discriminative which aims at differentiating the generated and true image.
Remark: use cases using variants of GANs include text to image, music generation and synthesis.
ResNet The Residual Network architecture (also called ResNet) uses residual blocks with a high number of layers meant to decrease the training error. The residual block has the following characterizing equation:
Inception Network This architecture uses inception modules and aims at giving a try at different convolutions in order to increase its performance through features diversification. In particular, it uses the $1times1$ convolution trick to limit the computational burden.
Part 0: Intro
Why
Deep Learning is a powerful toolset, but it also involves a steep learning curve and a radical paradigm shift.
For those new to Deep Learning, there are many levers to learn and different approaches to try out. Even more frustratingly, designing deep learning architectures can be equal parts art and science, without some of the rigorous backing found in longer studied, linear models.
In this article, we'll work through some of the basic principles of deep learning, by discussing the fundamental building blocks in this exciting field. Take a look at some of the primary ingredients of getting started below, and don't forget to bookmark this page as your Deep Learning cheat sheet!
FAQ
What is a layer?
A layer is an atomic unit, within a deep learning architecture. Networks are generally composed by adding successive layers.
What properties do all layers have?
Almost all layers will have :
- Weights (free parameters), which create a linear combination of the outputs from the previous layer.
- An activation, which allows for non-linearities
- A bias node, an equivalent to one incoming variable that is always set to
1
What changes between layer types?
There are many different layers for many different use cases. Different layers may allow for combining adjacent inputs (convolutional layers), or dealing with multiple timesteps in a single observation (RNN layers).
Difference between DL book and Keras Layers
Frustratingly, there is some inconsistency in how layers are referred to and utilized. For example, the Deep Learning Book commonly refers to archictures (whole networks), rather than specific layers. For example, their discussion of a convolutional neural network focuses on the convolutional layer as a sub-component of the network.
1D vs 2D
Some layers have 1D and 2D varieties. A good rule of thumb is:
- 1D: Temporal (time series, text)
- 2d: Spatial (image)
Cheat sheet
Part 1: Standard layers
Input
- Simple pass through
- Needs to align w/ shape of upcoming layers
Embedding
- Categorical / text to vector
- Vector can be used with other (linear) algorithms
- Can use transfer learning / pre-trained embeddings(see example)
Dense layers
- Vanilla, default layer
- Many different activations
- Probably want to use ReLu activation
Dropout
- Helpful for regularization
- Generally should not be used after input layer
- Can select fraction of weights (
p
) to be dropped - Weights are scaled at train / test time, so average weight is the same for both
- Weights are not dropped at test time
Part 2: Specialized layers
Convolutional layers
- Take a subset of input
- Create a linear combination of the elements in that subset
- Replace subset (multiple values) with the linear combination (single value)
- Weights for linear combination are learned
Time series & text layers
- Helpful when input has a specific order
- Time series (e.g. stock closing prices for 1 week)
- Text (e.g. words on a page, given in a certain order)
- Text data is generally preceeded by an embedding layer
- Generally should be paired w/
RMSprop
optimizer
Simple RNN
- Each time step is concatenated with the last time step's output
- This concatenated input is fed into a dense layer equivalent
- The output of the dense layer equivalent is this time step's output
- Generally, only the output from the last time step is used
- Specially handling for the first time step
LSTM
- Improvement on Simple RNN, with internal 'memory state'
- Avoid issue of exploding / vanishing gradients
Utility layers
- There for utility use!